Trading off performance and human oversight in algorithmic policy: evidence from Danish college admissions
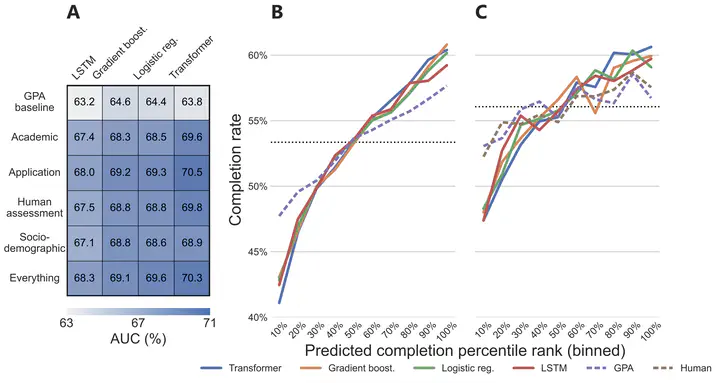
In our paper, we explore the potential of advanced AI models to improve decision-making in higher education admissions. Using a comprehensive dataset from Danish college admissions, we compare the effectiveness of sequential AI models against traditional methods based on high school GPAs or human judgment.
Our results demonstrate that AI models offer more accurate and fair predictions of student dropout rates compared to simpler models, even when those models consider protected sociodemographic factors. Interestingly, the AI-driven approach also reveals that certain student profiles are better suited for specific programs, highlighting opportunities for policy-driven efficiency gains.
Given the recent Danish nationwide policy to reduce admissions by 10%, we estimate that leveraging AI for admission decisions could lead to significant economic benefits. However, this efficiency comes at a cost: the potential reduction of human oversight and transparency in the admissions process.
Our work underscores the delicate balance policymakers must strike between optimizing performance and maintaining accountability when deploying algorithmic systems in public policy.